Revolutionizing Seismic Horizon Interpretation with AI
In the subsurface reservoir characterization for oil and gas exploration, development planning and CO2 storage assessment, the interpretation of geological horizons from 2-D & 3-D seismic data stands as a critical cornerstone, profoundly influencing the quality of reservoir modeling, reserve estimate and development planning. Mapping reservoir horizons, central to seismic interpretation projects, involves a detailed analysis of seismic reflections to map the subsurface stratigraphy, crucial for identifying potential reservoirs and understanding geological structures. Despite its significance, this process is notoriously time-consuming, especially in reservoir zones where seismic data quality is often compromised. Challenges such as inconsistent seismic reflection patterns along the same geological horizons, which may not align with the anticipated peak or trough signals, add layers of complexity, making accurate interpretation a formidable task in both conventional and unconventional plays.
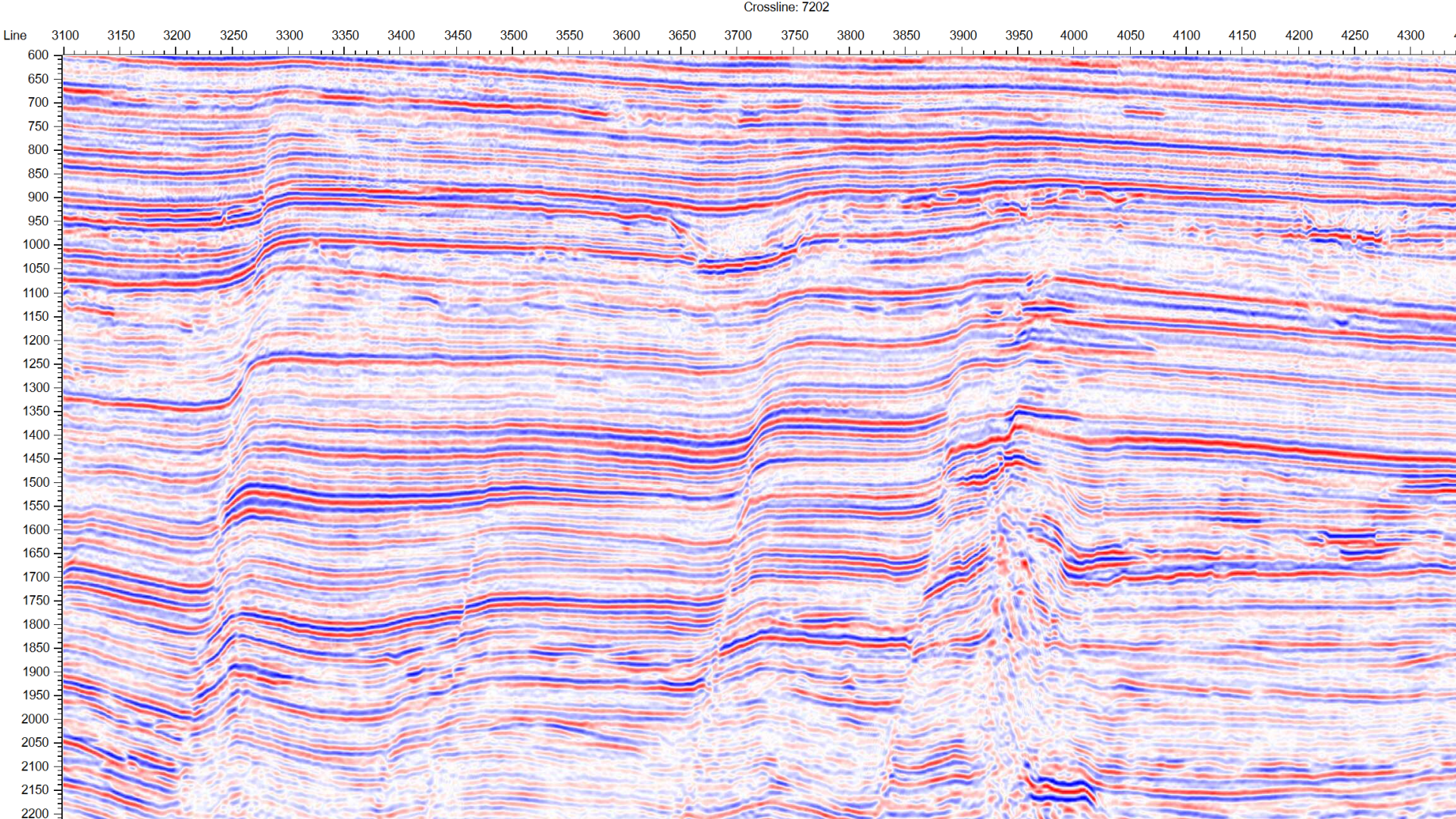
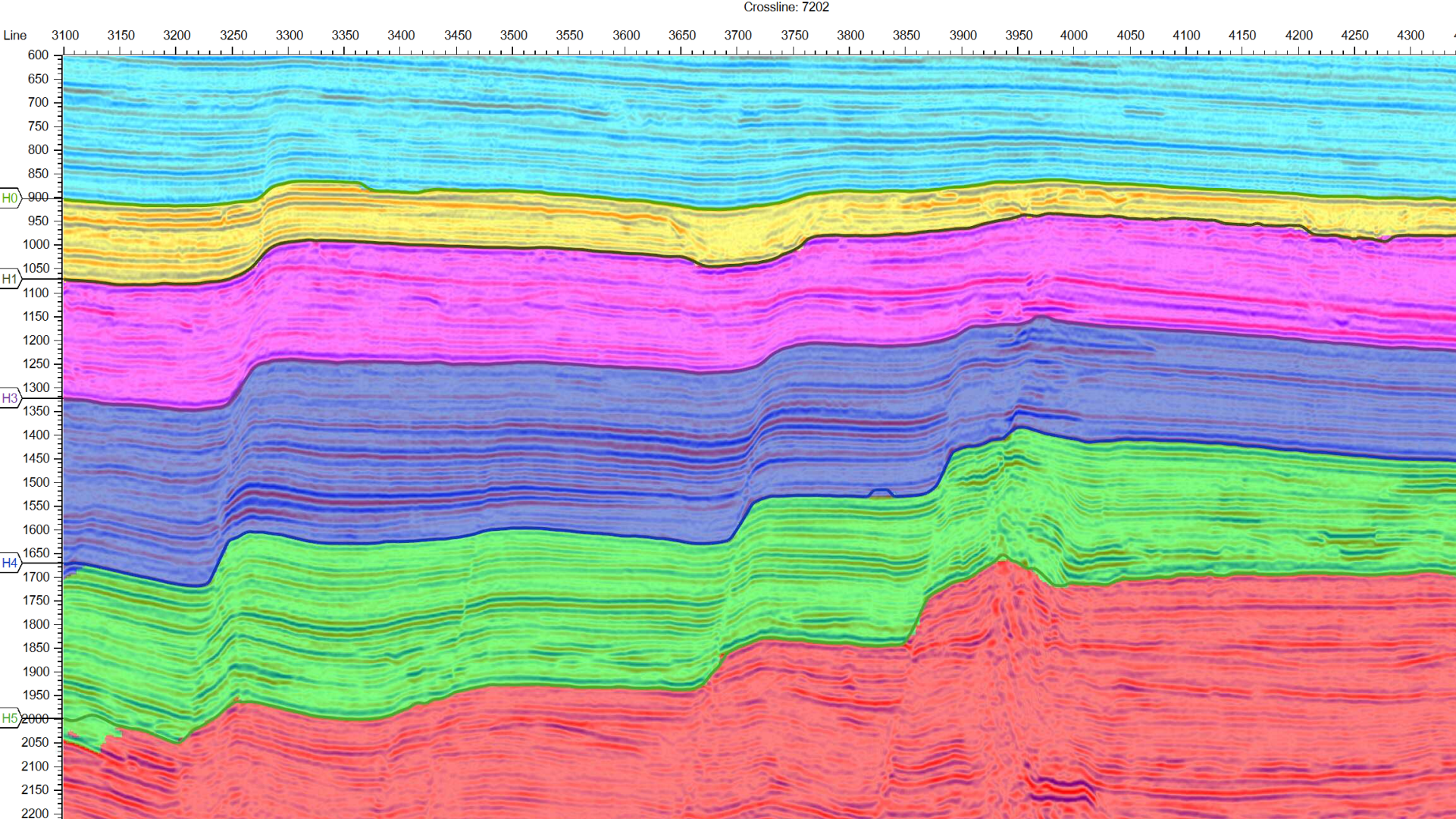
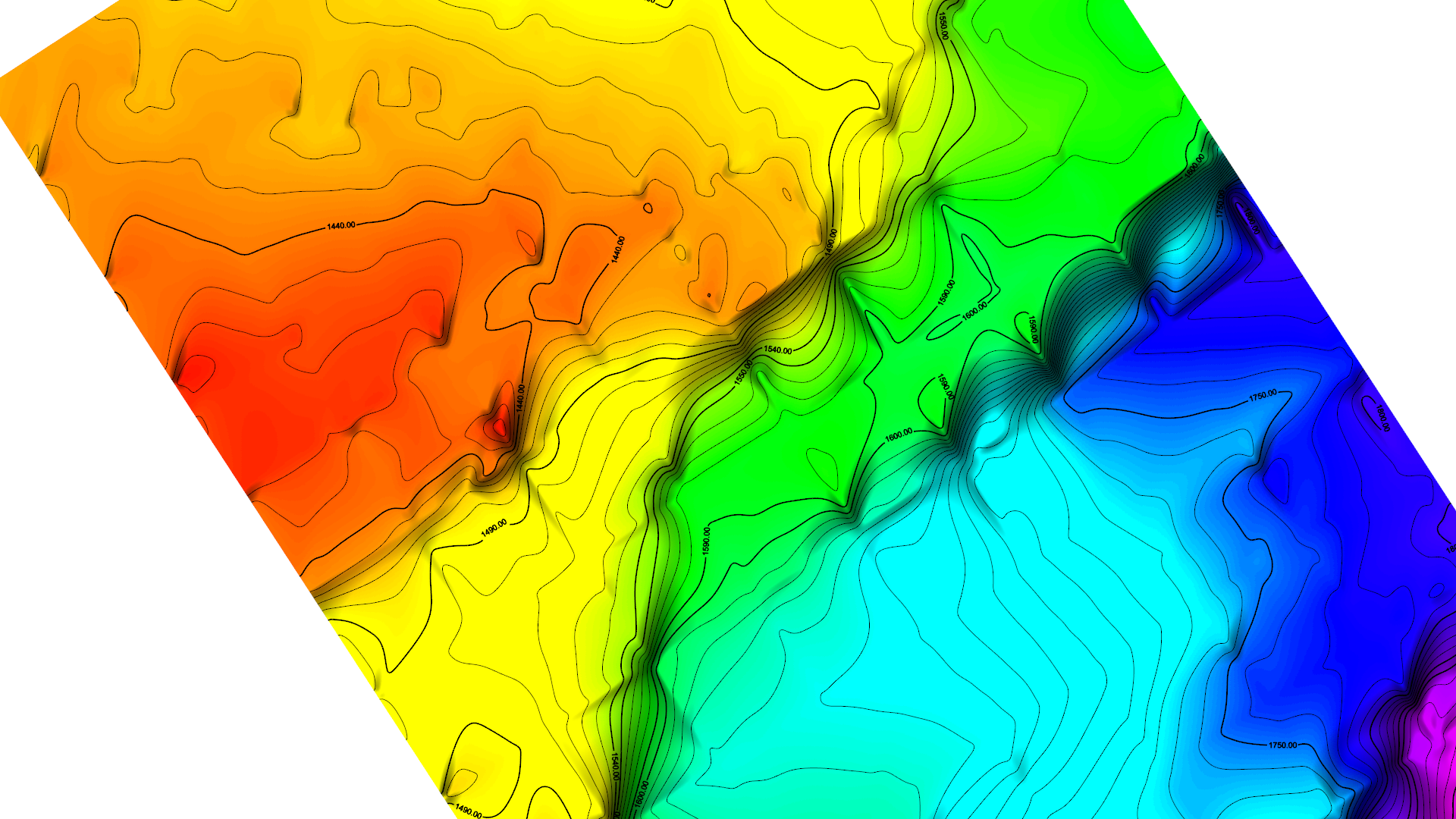
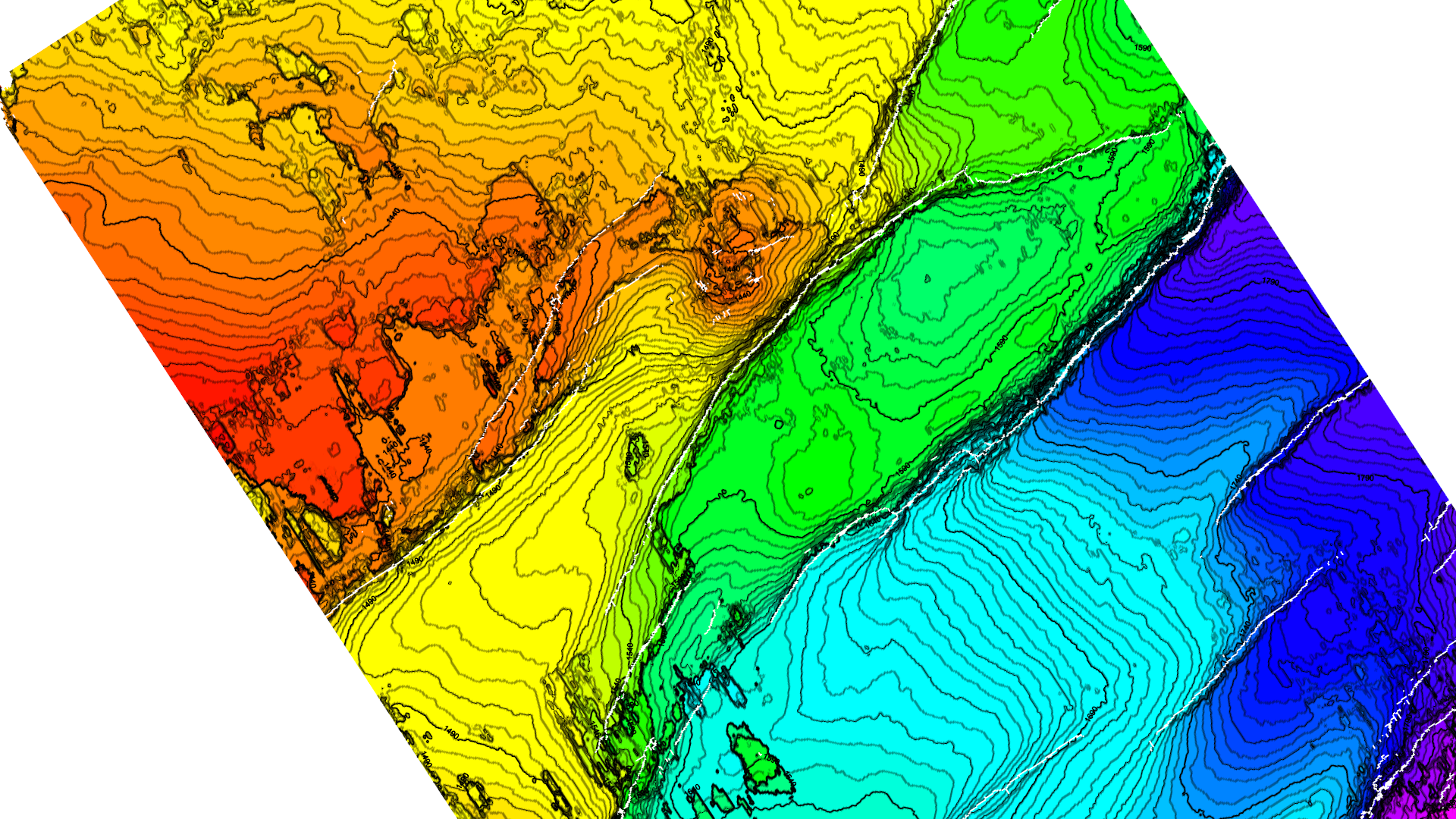
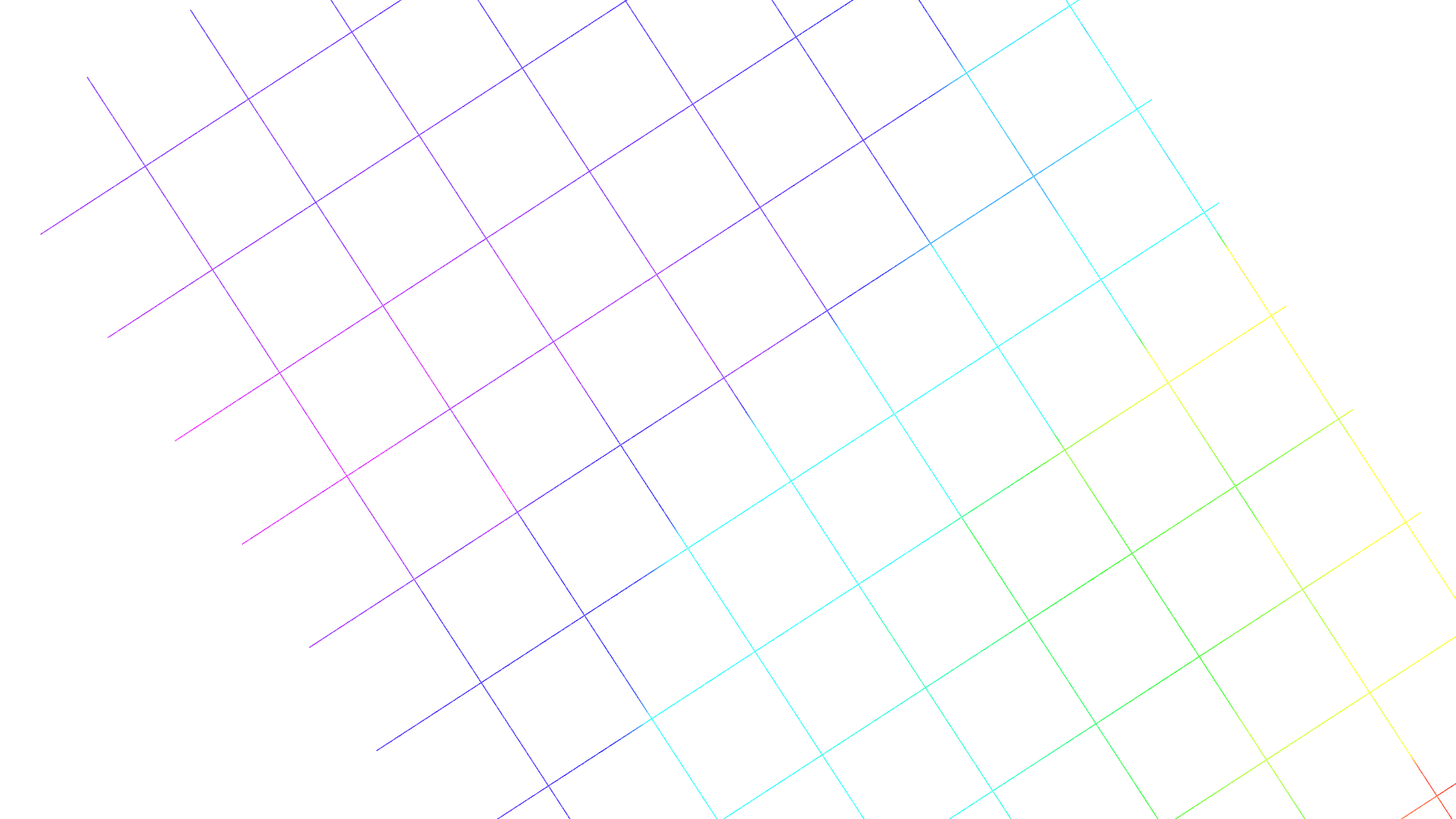
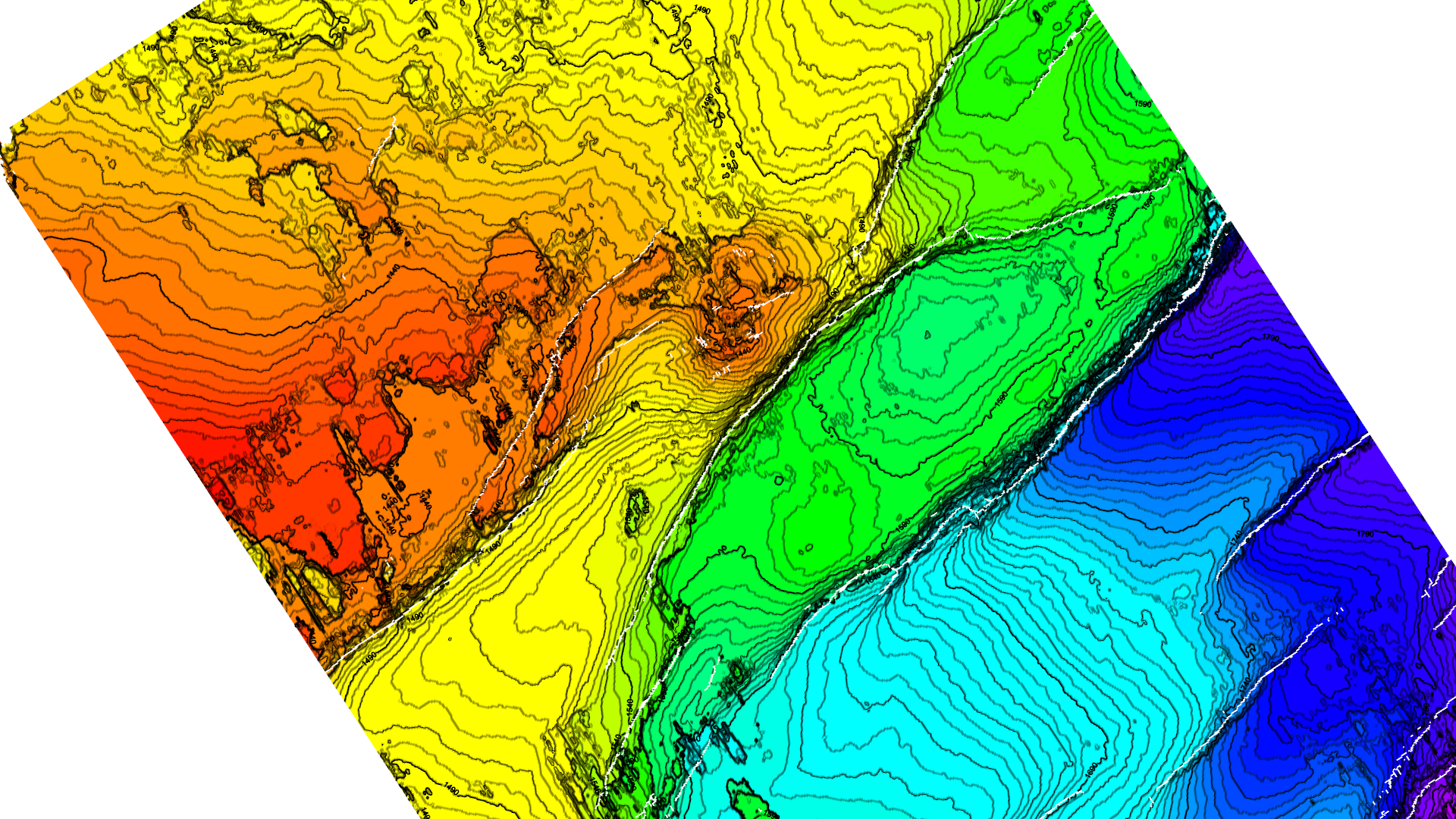
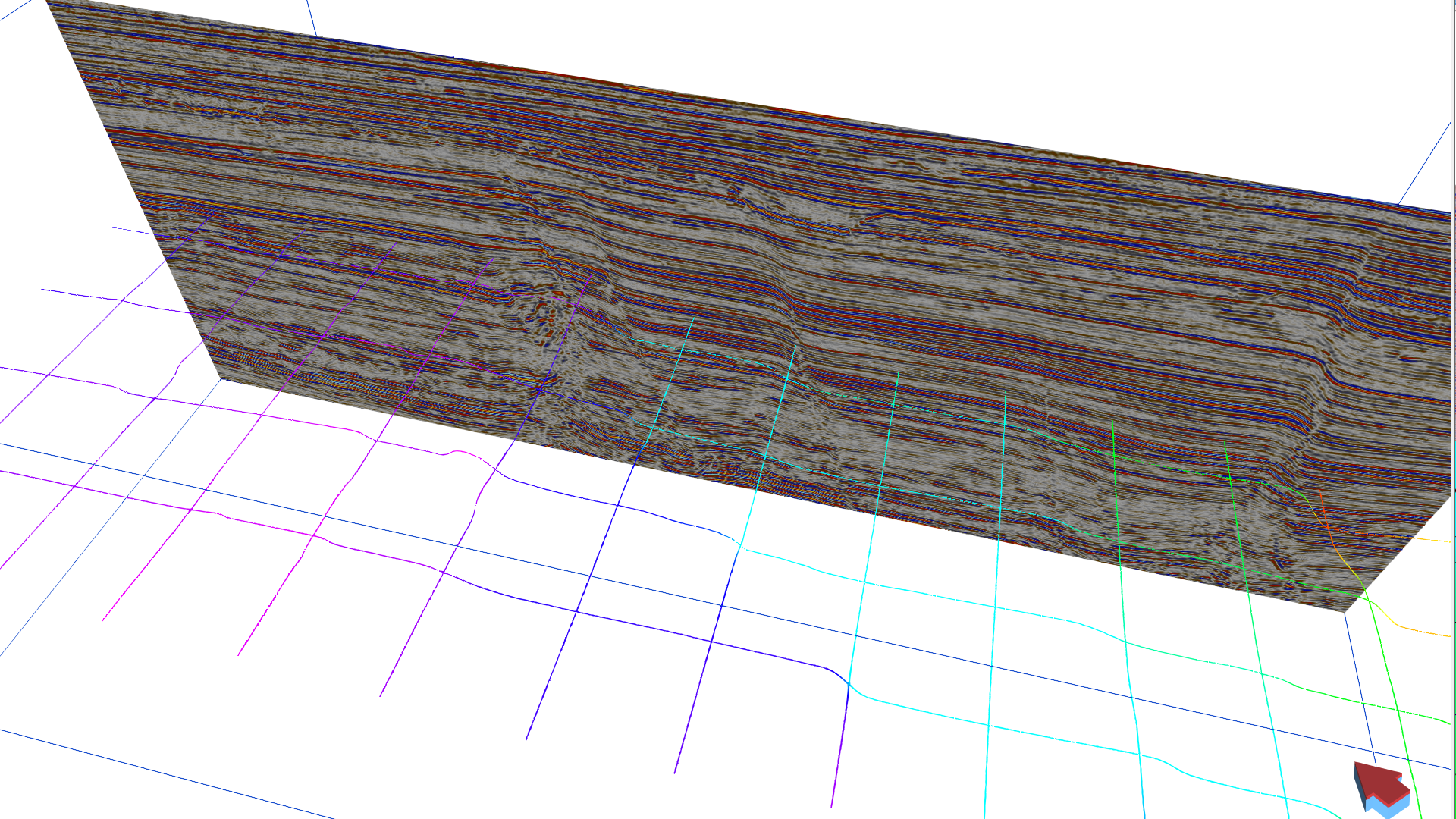
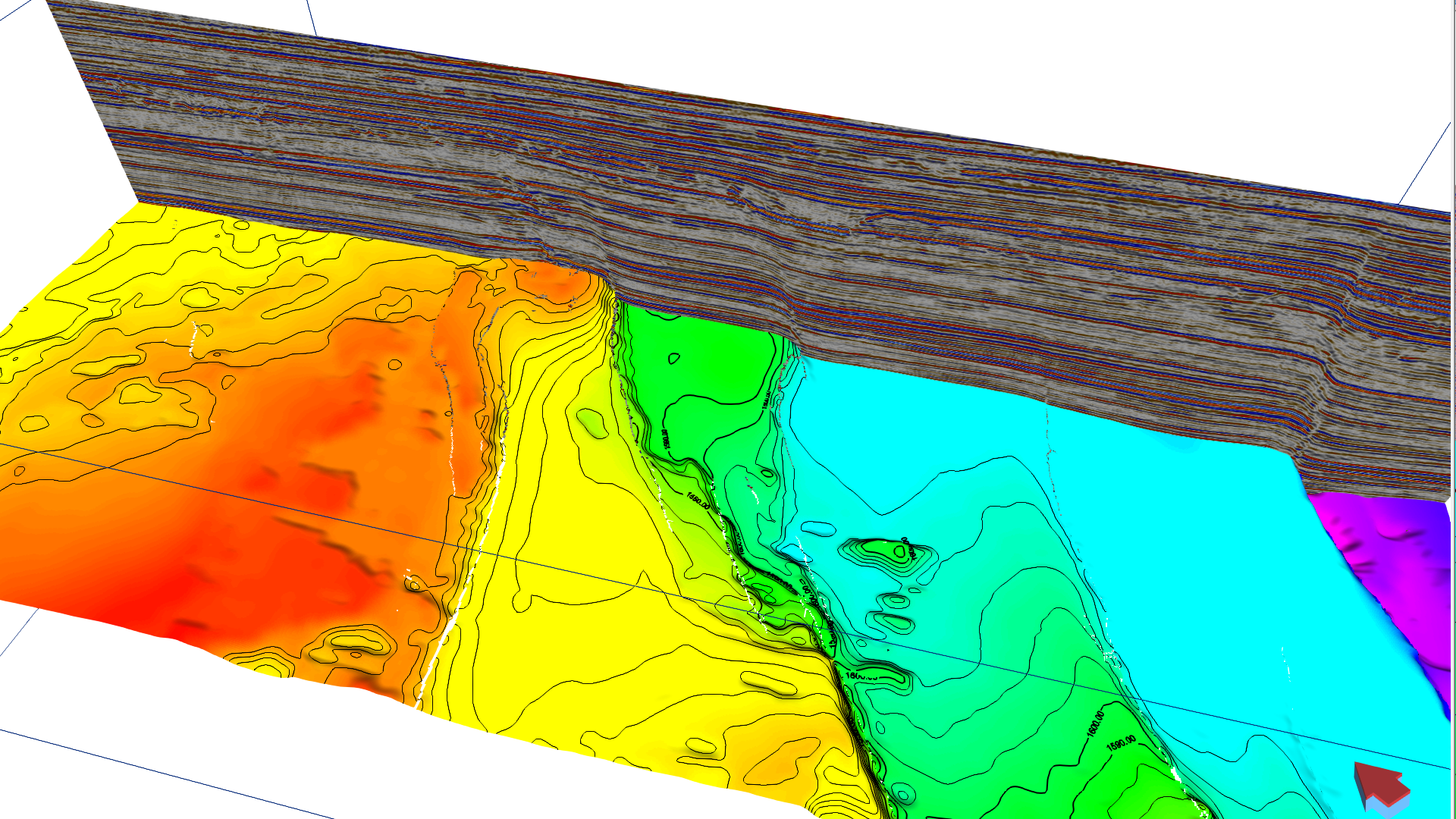
Traditionally, these challenges have necessitated a reliance on 2-D manual interpretation methods of 3-D seismic volumes, a painstaking and labor-intensive process. This approach is significantly hampered by the limitations of traditional horizon 3-D tracking methods, which struggle in the presence of faults and noise. These traditional algorithms are largely based on the local correlation of waveform signatures within a specific window, contrasting sharply with the holistic approach taken by human interpreters who evaluate seismic reflection patterns over large areas, often encompassing the entire seismic section.
The evolution of Artificial Intelligence (AI) technology has introduced a groundbreaking solution to these longstanding challenges. AI algorithms are now capable of emulating the sophisticated process of human interpretation, recognizing complex reflection patterns of seismic sequences, and accurately tracking the boundaries of these sequences as horizons. This capability enables the comprehensive interpretation of all horizons within 3-D volumes or a in a 2-D survey that consists of many 2-D sections, marking a significant advancement in seismic interpretation for both 2-D and 3-D seismic data.
AI’s transformative impact in seismic horizon interpretation is underpinned by its ability to leverage training data derived from key sections interpreted by geophysicists. This ensures that the AI-interpreted horizons are both accurate and respectful of the interpretations provided by experts, integrating human expertise with the computational power of AI. This novel approach contrasts markedly with traditional interpretation methods, which necessitate exhaustive manual picking across sections. AI-assisted workflows, however, require interpreting less than 1% of key sections as training data, leading to an exponential productivity gain of more than tenfold.
Furthermore, the precision and detail achieved by AI in interpreting seismic horizons far surpass traditional manual interpretations and their resultant horizon maps, which often lose vital geological details through interpolation. AI’s ability to capture and analyze intricate seismic patterns, recognized by geophysicists during the training phase, ensures that every nuance between training sections is meticulously represented. This level of detail not only enhances the accuracy of seismic interpretations but also deepens our understanding of the reservoir architecture.
The integration of AI into seismic horizon interpretation extends beyond mere efficiency gains, heralding a new era in subsurface reservoir characterization and modeling. This technological leap enables geoscientists to focus their expertise on more advanced analytical tasks and strategic decision-making. The acceleration in subsurface horizon mapping from seismic data facilitated by AI is particularly critical in the energy sector and CO2 storage reservoir assessment, where the precise delineation of subsurface geology can uncover new resources and optimize extraction methods.
Moreover, the enhanced interpretative capabilities provided by AI promise to unlock new insights into the subsurface, revealing previously undetected features and contributing to a more comprehensive understanding of reservoirs. These advancements are not only pivotal for resource exploration but also hold immense potential for environmental monitoring, hazard assessment, and geological risk mitigation for CO2 storage.
As AI technologies continue to evolve and mature, their impact on seismic interpretation and subsurface data integration is poised to grow exponentially. The integration of AI marks a significant shift towards more efficient, accurate, and insightful subsurface mapping and modeling methodologies. This journey toward harnessing AI in the service of subsurface exploration is akin to navigating the uncharted depths of subsurface reservoirs, where each discovery brings us closer to unraveling its mysteries.
In conclusion, the advent of AI in the field of seismic horizon interpretation represents a paradigm shift, offering unprecedented efficiency, accuracy, and depth of insight. This revolution in the subsurface exploration not only enhances our understanding of the subsurface reservoirs but also opens new frontiers in the quest for natural resources, environmental stewardship, and better selection of CO2 storage locations. As we continue to explore these depths with AI as our guide, the future of subsurface exploration and reservoir characterization shines brighter than ever, promising a new era of discovery and innovation that will reshape our interaction with the complex process of oil and gas exploration and production, and CO2 geologic storage.