Revolutionizing Fault Detection
Subsurface Reservoir Characterization with InterpretationAI
Accurately mapping the spatial distribution of faults and fractures is paramount in subsurface reservoir characterization. This holds especially true for unconventional plays, where understanding the fault framework is not merely an academic exercise—it is critical to mitigating drilling risks and minimizing the potential for induced seismicity.
Traditional seismic attributes have long been the mainstay for delineating fault patterns. Attributes like semblance and coherence are adept at calculating waveform differences across a moving window of seismic samples, highlighting areas of discontinuity. However, these conventional methods have their drawbacks. They do not discriminate between waveform discontinuities caused by faults and those resulting from other stratigraphic or depositional features such as the boundary of pitchout, channel boundaries, and various geobodies. As such, lineament features indicated by coherence and similar seismic attributes require careful interpretation by geoscientists, leaving room for subjectivity.
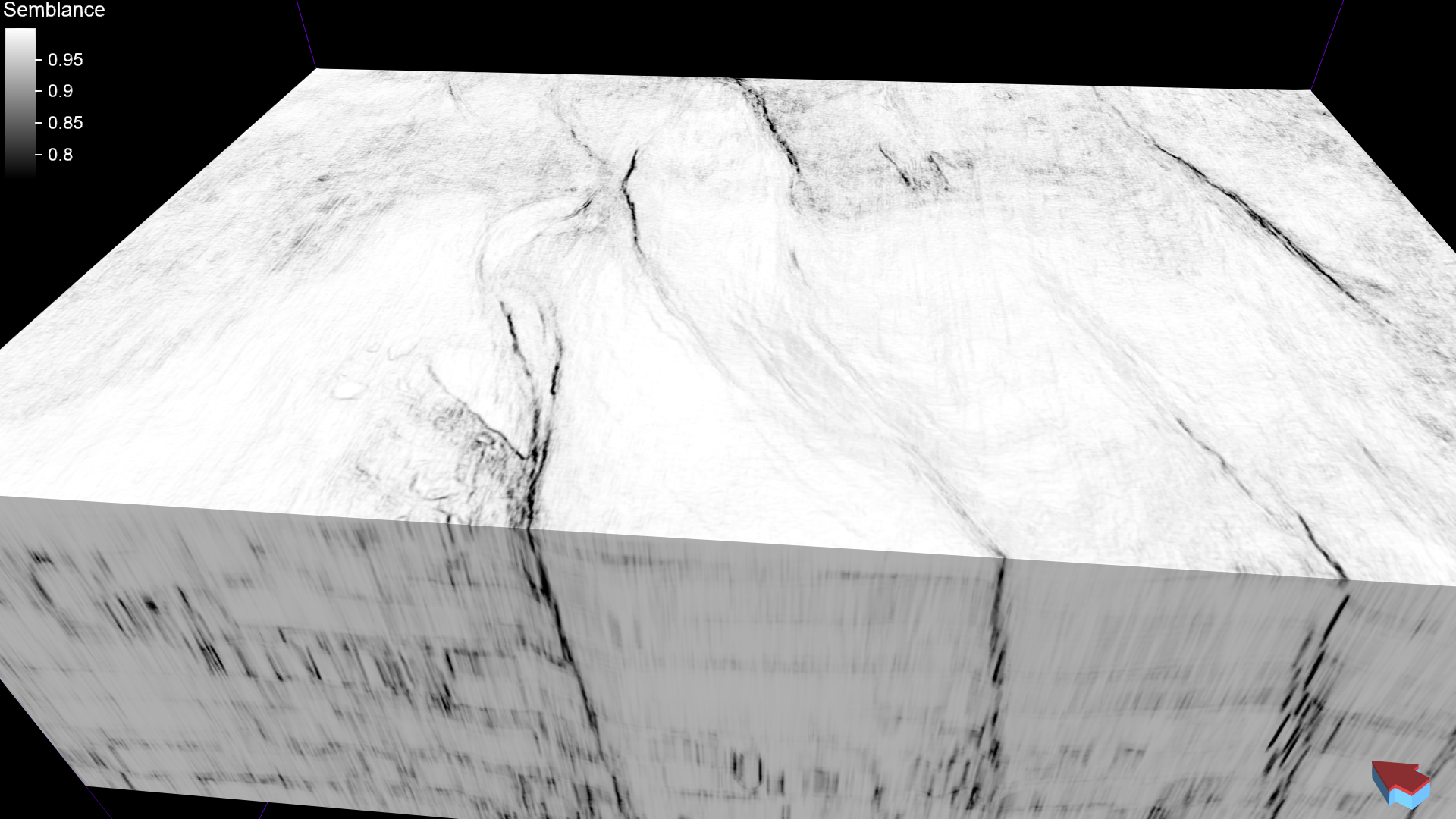
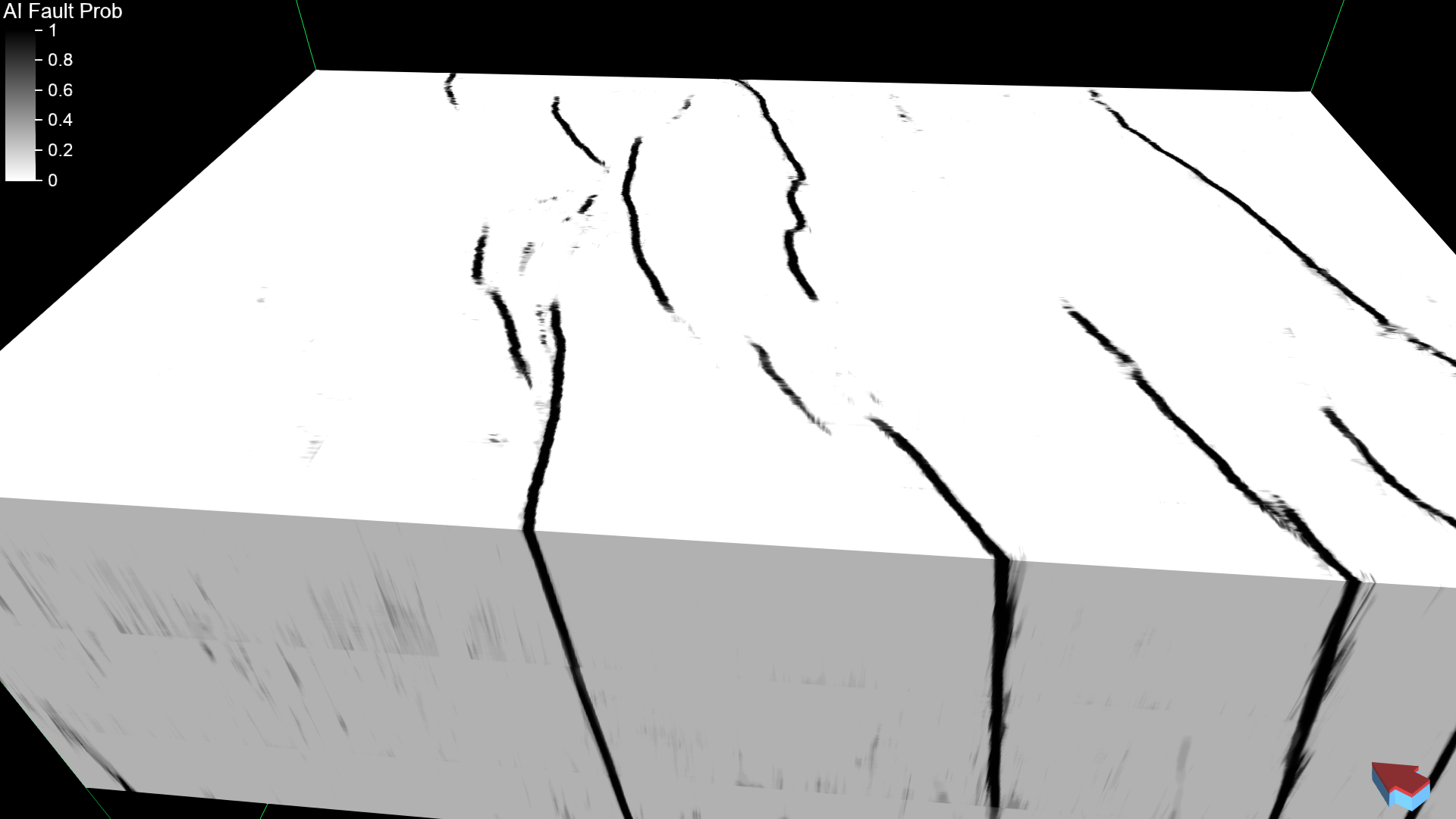
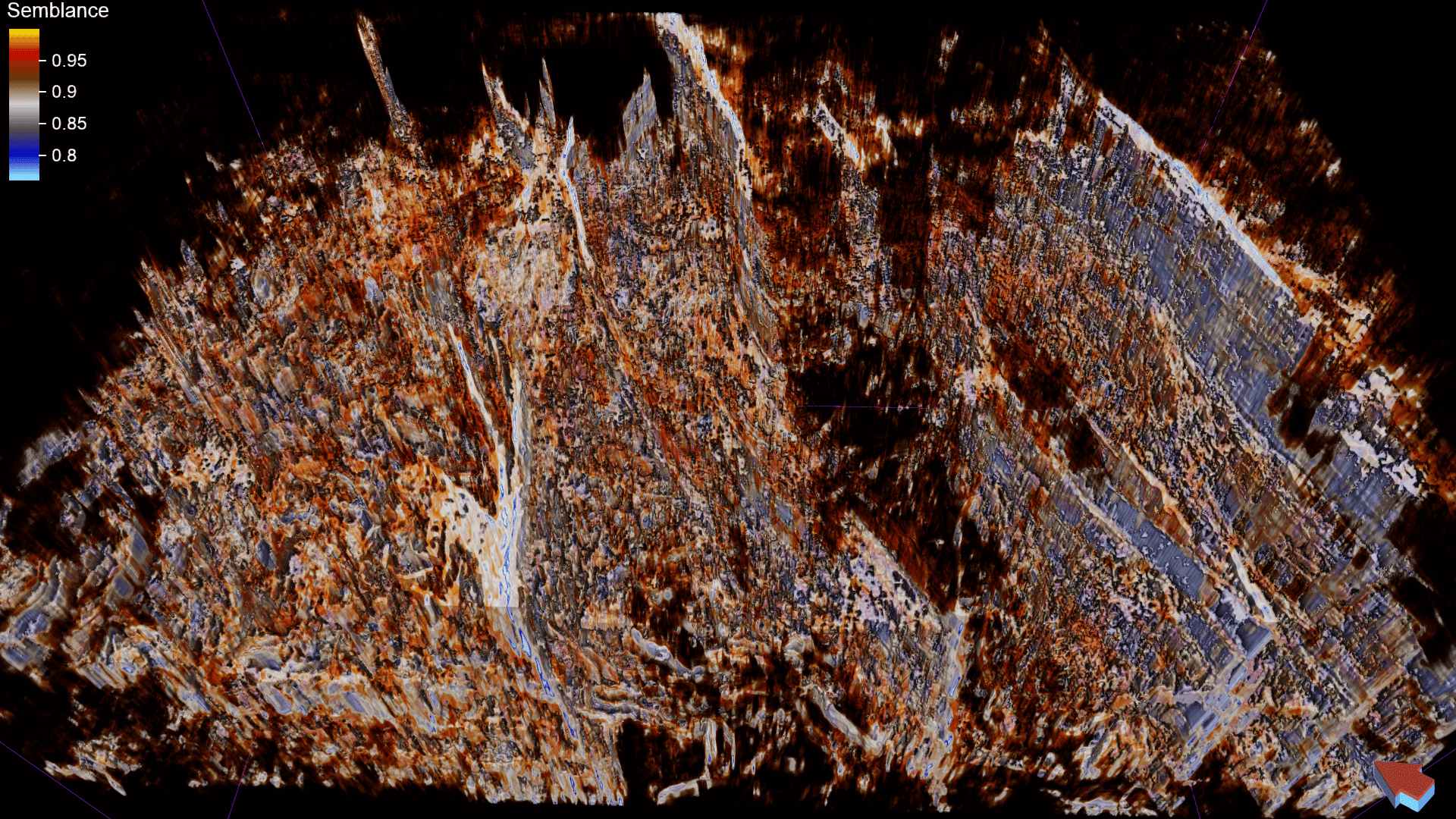
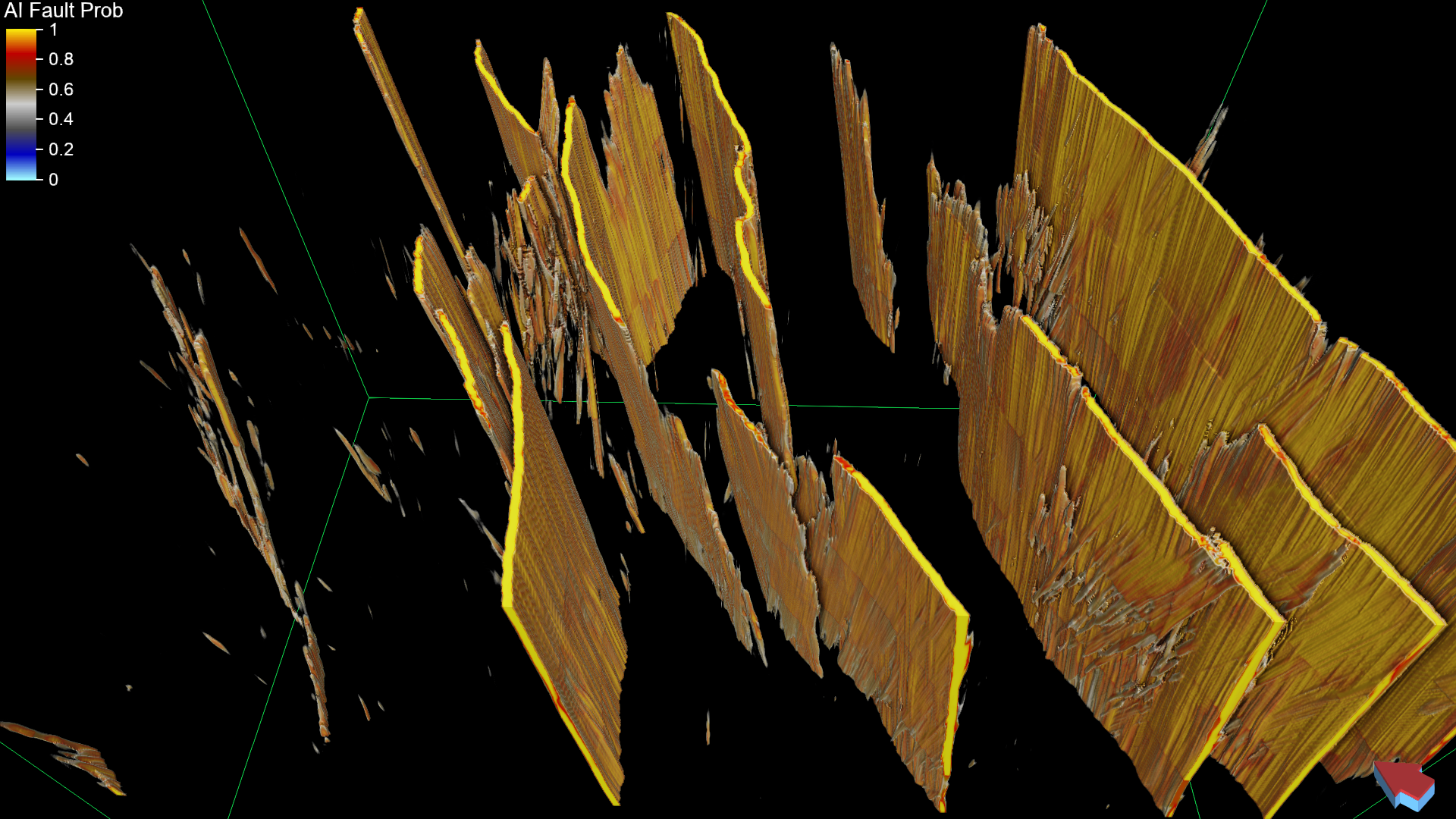
Another challenge with conventional seismic attributes is their sensitivity to noise. Such noise can compromise the accuracy of fault detection, often resulting in ambiguous attribute responses that can mislead the interpretation process.
interpretationAI, introduces an evolutionary method for calculating fault occurrence probability (ranging from 0 to 1) at every seismic sample. It achieves this by leveraging a deep-learning model trained from geoscientists’ interpretations on select seismic sections. The result is an AI model that encapsulates the interpretation expertise of seasoned geoscientists, providing a fault volume that aligns with human interpretation and sidesteps the “false positives” commonly associated with AI models trained on synthetic seismic data.
interpretationAI further enhances productivity with an innovation function for auto-tracking of fault surfaces and fault sticks directly from fault probability volumes. What once took weeks can now be accomplished in hours—you can map all faults from a vast seismic volume with unprecedented speed and accuracy.
The figures accompanying this text vividly demonstrate the difference between conventional fault attributes and AI-driven fault probabilities. They showcase the clarity with which InterpretationAI can delineate faults, offering a compelling visual affirmation of its superior detection capabilities.
In conclusion, interpretationAI is not just another tool—it’s a transformative force in fault mapping, rendering previously complex and time-consuming processes both efficient and reliable. Embrace the future of subsurface exploration with InterpretationAI, where AI meets the expert’s intuition to unlock the full potential of seismic data.